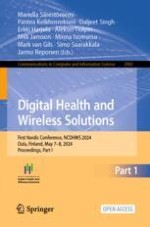
Open Access 2024 | OriginalPaper | Buchkapitel
How Does Human-Centred Extended Reality Support Healthcare Students’ Learning in Clinical Conditions?
verfasst von : Kristina Mikkonen, Hany Ferdinando, Marta Sobocinski, Heli Kuivila, Sari Pramila-Savukoski, Tugba Vhitehead, Paula Ropponen, Teemu Myllylä, Jari Paunonen, Erson Halili, Joel Koutonen, Juha-Matti Taikina-Aho, Antti Siipo, Sanna Järvelä
Erschienen in: Digital Health and Wireless Solutions
Verlag: Springer Nature Switzerland
Aktivieren Sie unsere intelligente Suche, um passende Fachinhalte oder Patente zu finden.
Wählen Sie Textabschnitte aus um mit Künstlicher Intelligenz passenden Patente zu finden. powered by
Markieren Sie Textabschnitte, um KI-gestützt weitere passende Inhalte zu finden. powered by