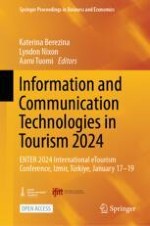
Open Access 2024 | OriginalPaper | Buchkapitel
UGC-Based Factors Influencing Customer Satisfaction Pre and Post COVID-19: The Case of Lake Constance
verfasst von : Dominic Regitz, Wolfram Höpken, Matthias Fuchs
Erschienen in: Information and Communication Technologies in Tourism 2024
Verlag: Springer Nature Switzerland
Aktivieren Sie unsere intelligente Suche, um passende Fachinhalte oder Patente zu finden.
Wählen Sie Textabschnitte aus um mit Künstlicher Intelligenz passenden Patente zu finden. powered by
Markieren Sie Textabschnitte, um KI-gestützt weitere passende Inhalte zu finden. powered by